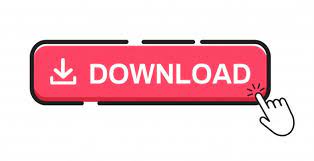

Indicate the balance between fitness and simplicity of the model. InĪddition to Mallows' CP, JMP shows Akaike's information criterion correction (AICc) to The process is so interactive that the analyst canĮasily determine whether certain variables should be kept or dropped. Predictors, JMP (SAS Institute, 2010) allows the user to select or deselect Use NCSS (NCSS Statistical Software, 1999).Īlthough the result of stepwise regression depends on the order of entering Perform a variable selection in SAS, the syntax is "PROC REG MODEL R-square, Root Mean Square Error, and Mallow's Cp are different, theĬonclusion is the same: One is too few and three are too many. Nevertheless, although the approaches of maximum
SAS JMP INFLUENCE COOKS D FULL
RMSE of the best two-variable is 1.81 but that of the full model isġ.83 (see the red arrow in the right panel)! The Cp of the best two isĢ.70 whereas that of the full model is 4.00 (see the red arrow in the RMSE and Cp, the full model is worse than the two-variable model. But the curve turns into flat from two to three (see the red It clearly indicates a sharp jump from one to The x-axis represents the number of variables while the y-axis If you cannot follow the above explanation, this figure may help you. However,įrom the two-variable to the full model, the gain is trivial (.62. Last, all three variables are used for a full model (R 2=.62).įrom the one-variable model to the two-variable model, the varianceĮxplained gains a substantive improvement (.60. In all one-variable models, the best variable is X 3 according to the max. The following output is based on a hypotheticalĪt first, each regressor enters the model one by one. The principle illustrated here can be well-applied to the situation of The lower the RMSQ and Cp are, the better the model is.įor the clarity of illustration, I use only three regressors: X 1, X 2, X 3. Thus, the higher the R-square is, the better the model Mallow's CP is the total square errors, as opposed to the best fit by RMSE is a measure of the lack of fit while N-models based upon the largest variance explanied. R-square is a method of variable selection by examining the best of Maximum R-square, Root Mean Square Error (RMSE), and Mallow's Cp. There are other better ways to perform variable selection such as

Thus, we can assert that X 1 and X 4 are more influential to the dependent variable than X 2 and X 3. To the variance explained by each variable to the model is clearly In the right panel we have a "clean" model. Importance of independent variables if all predictors are orthogonal. However, weĬan interpret the result of step regression as an indication of the "importance" depends on the selection order (Bring, 1996). Indeed, the more correlated the regressors are, the more their ranked Seems to be less influential because its contribution to the varianceĮxplained has been overlapped by the first variable, and X 3 and X 4 are even worse. If X 1 enters the model first, it seems to contribute the largest amount of variance explained. We cannot tell which variableĬontributes the most of the variance explained individually. In the left panel X 1-X 4Īre correlated (non-orthogonal). Assume that there is a Y outcome variable and four regressors X 1-X 4. Regressors with some degree of correlation would return inaccurate Predictors are independent (But if you write a dissertation, it doesn't However, the above interpretation is valid if and only if all Predictors by its magnitude of influence on the outcome variable (e.g. Many researchers employed these techniques to determine the order of There are several versions of stepwise regression such as forward selection, backward elimination, and stepwise. Variable that cannot contribute much to the variance explained would be A stepwise regression is a procedure toĮxamine the impact of each variable to the model step by step. One common approach to select a subset of variables from a complex When there are two observations, the regression line is a Is one subject only, the regression line can be fitted in any way (leftįigure). The following cases are extreme, but you will get the idea. Of observations, this model is said to be lack of degree of freedom and the number of parameters to be estimated is larger than the number In addition, when there are too many variables in a regression model Inevitably many of those independent variables will be too correlated. As what IĮxplained in my example of "fifty ways to improve your grade, " Mistake is to put too many regressors into the model. The problem of too many variables Stepwise regressionĬollinearity happens to many inexperienced researchers.
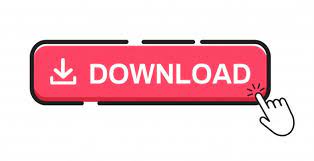